Introduction
In today’s world, data breaches are a fact of life for both consumers and companies. It’s become somewhat of a truism to point out that for many companies breaches are a matter of if not when as defenders are at a significant disadvantage. The reason this is the case is that over the past 15+ years, we’ve seen the growth of a concerning trend that’s become almost banal today – the rise of what has been dubbed “mega-breaches.” This term is used to refer to breaches impacting 1 million or more records, which was once upon a time a startling hallmark. The first of these breaches occurred in the summer of 2004, when an AOL engineer exfiltrated 92 million screen names to sell to scammers. This incident is believed to have impacted at least 30 million customers. At the time, such a crime was so rare that the judge initially wasn’t sure how to sentence the perpetrator and refused his initial plea bargain. Since then, data breaches have only continued to balloon in scope, with mega-breaches becoming more prominent.
In order to investigate how the trend of mega-breaches has taken shape over the last 15+ years, we took a look at the top 100 breaches between 2004 and 2020, ranked by the number of records impacted. Based on our analysis, we found that on average mega-breaches increased 36% year over year. After 2016, data breaches impacting more than 500 million records became more frequent. In 2020, yet another milestone was reached when multiple breaches impacting billions of records occurred. From our analysis, it was clear that not all mega-breaches were created equal. Because of the frequency and size of mega-breaches, it makes sense to develop a new way of thinking about these incidents. To that end we looked at a variety of metrics for these incidents, such as:
- The industry of the company impacted by the incident
- The number of records and where possible the number of individuals impacted by the incident
- The cause of the incident as well as the attack vectors used to exfiltrate data and the systems where the data was stored if applicable
- The time to discovery and disclosure for the incident
- The cost of the incident
- The types of records exposed as a result of the incident
Highlights
Some of the highlights we found include:
- On average, the incidents in the top 100 breaches impacted 147.2 million individuals per year.
- 52% of the incidents analyzed were the result of system misconfigurations causing a data leak, or involved a threat actor actively exploiting such a misconfiguration.
- 30% of the incidents analyzed exposed password hashes. 16% of these incidents also included passwords hashed with weak encryption (SHA-1, MD5 or something similar) or plain text/cleartext passwords.
- In total, the incidents we analyzed cost at minimum a combined 8.8 billion dollars and exposed 51 billion records.
- The average time to discovery for an incident on this list was 62 weeks and the average time to disclosure to the public was 78 weeks and 5 days.
For additional highlights, see our infographic down below.
List of the top 100 largest breaches of the 21st century (2004-2020)
Methodology
As stated above, we looked at the 100 largest reported data breaches from 2004 to 2020 by number of records exposed or by number of individuals affected. In eight instances we could not verify the number of individuals affected, so we recorded the number of records exposed but did not provide a value for the number of individuals impacted. In cases where we could only find the number of individuals affected, we made the conservative assumption that the number of records exposed was at least equal to the number of individuals impacted by the breach.
We define a record as a piece of information that can either be associated with a single user – like a name or IP address – or in some cases information that can be associated with an organization’s internal systems. This definition is important, as records breached and individuals impacted can sometimes be conflated in media reporting of breaches. For example, in the 2004 AOL story we mentioned above, while there were 92 million screen names stolen in breach, at the time AOL had only about 30 million or so users, with some users having duplicate screen names.
To find these incidents we used public sources like Privacy Rights Clearinghouse and similar publicly managed data breach datasets. The list we created was cross-referenced with other public sources, including news stories to verify facts like the number of individuals impacted and where possible additional information like costs incurred by the affected organization and how long it took them to discover the incident. We also used news reports to codify incident causes. We excluded incidents in which reporting conflicted on the number of individuals or records impacted or where we felt like enough information hadn’t been provided to ascertain the cause or scope of the incident.
Causes are broken down into three parts for every incident:
- By threat actor – We used the terms insider threat or external threat actor to denote whether an organizational insider or someone outside the organization is known to have caused the breach. We used system misconfiguration as a third category for accidental exposures or “data leaks” where no threat actor is believed to have accessed the data.
- By attack vector – This secondary description elaborates on how the threat actor or exposure event occurred. In the instance that reporting on a particular incident was limited or the victim organization did not disclose the attack vector “unknown/undisclosed” is the given description.
- By system – In the instance the systems where the data was taken from are known we included them as a tertiary description. These were mostly only disclosed in instances of system misconfigurations. If the cause was unknown, we left the field blank.
Summary of Findings
Top 100 largest data breaches over time
We first visualized how the top 100 breaches were distributed over the 16 years we looked at, as seen in Figure 1 – Top 100 breaches by Year 2004-2020. These breach events are disproportionately represented later in the decade, starting to rise dramatically in 2013 with a 133% increase. The number of mega-breach events peaks in 2018 at 21 events which is a 250% increase from the previous year.
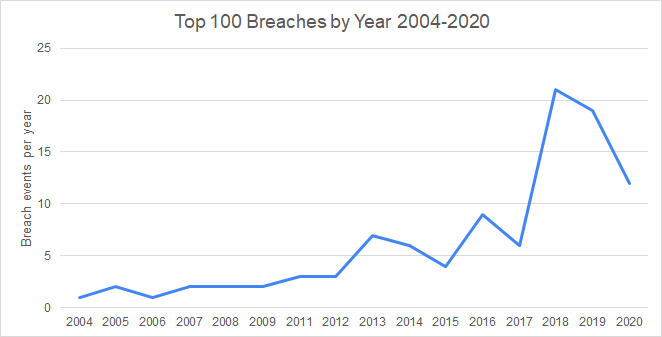
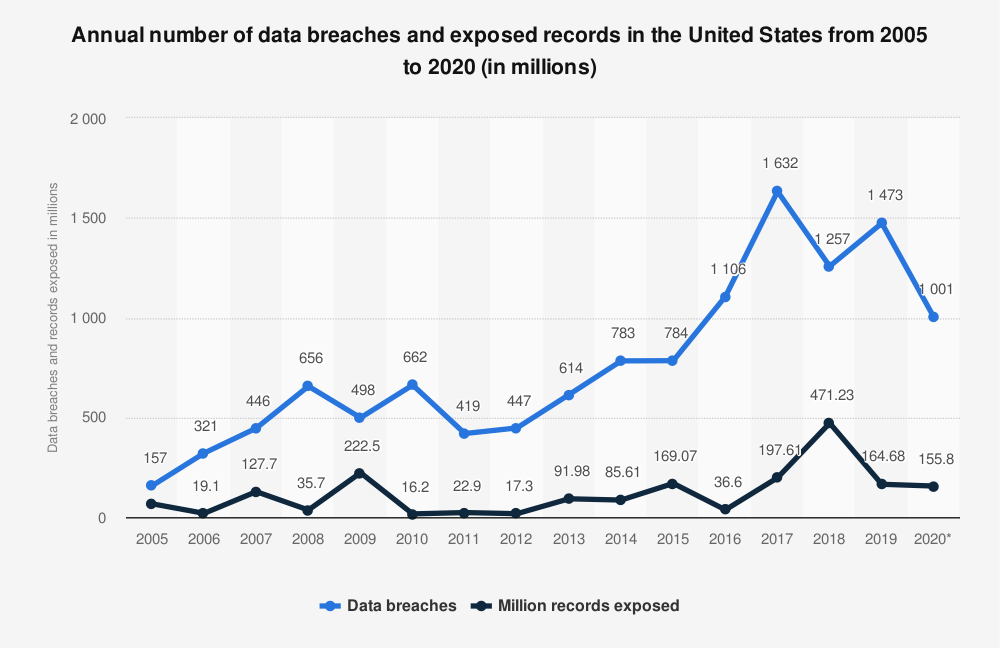
Although our dataset is not a random sample, the distribution of the top 100 breaches falls roughly in line with reporting and analysis on breaches as a whole. For example, the Identity Theft Resource Center which has been reporting on breaches for more than 15 years, shows a somewhat similar trajectory for the growth of data breaches over time. We found that the vast majority of mega-breaches analyzed occurred in years when data breaches overall were on the rise. Figure 2 – Annual number of data breaches and exposed records in the United States from 2005 to 2020 uses data collected from the ITRC to illustrate the marked growth in data breaches starting around 2012 and peaking around 2017 before dropping off afterwards.
It’s worth noting that the ITRC’s inclusion methodology for data breaches differed from ours, so several of the mega-breaches in our analysis do not factor into their graph of the number of records exposed over time. This is worth keeping in mind when looking at our next graph.
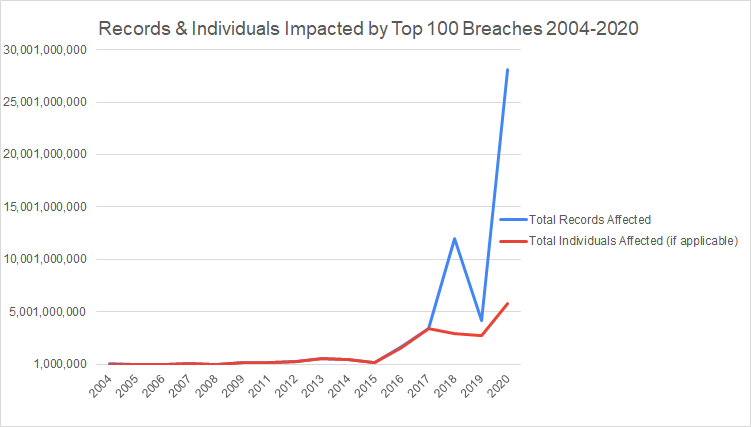
To get a sense of the impacts of mega-breaches over time, we looked at the total number of records exposed and individuals affected from 2004 to 2020. For over 80% of incidents we either found the number of records and individuals affected to be the same or assumed they were as reporting for many incidents tended to solely focus on the number of individuals affected. Even still, there were a handful of breach incidents in which the number of records exposed varied wildly from the number of individuals or customers affected. For example, the 2018 Apollo data leak which resulted from a misconfigured AWS web server exposed some 9 billion unique data points on companies and individuals but only affected around 212 million customers.
The divergence between records and individuals is sharpest in 2020, in part because of the disturbing trend of mega-breaches consistently reaching upwards of 1 billion records exposed. Of the top 100 data breaches, seven involve incidents where 1 billion or more records were exposed. Four of these (57%) occur in 2020 which indicates a smaller number of incidents were disproportionately responsible for an increasingly large proportion of records exposed.
The vast majority of these billion-record data breaches are better thought of as data leaks or “mega-leaks” as they don’t involve threat actors breaching a perimeter. Instead, these incidents occur when a system (usually a cloud database) becomes internet-facing by accident. For many of these incidents, the number of individuals affected went unreported, which also accounts for the wide divergence shown on the graph. This is a trend we discussed in our Securing Best of Breed SaaS Apps webinar near the beginning of 2021, which you can see in the clip below.
https://www.youtube.com/watch?v=JGEGDJUhLT8&list=PLDML4npVucybjeCmiGZdY0GFfZgWcdSCt&index=1
Types of Records Most Commonly Exposed in the Top 100 largest Breaches
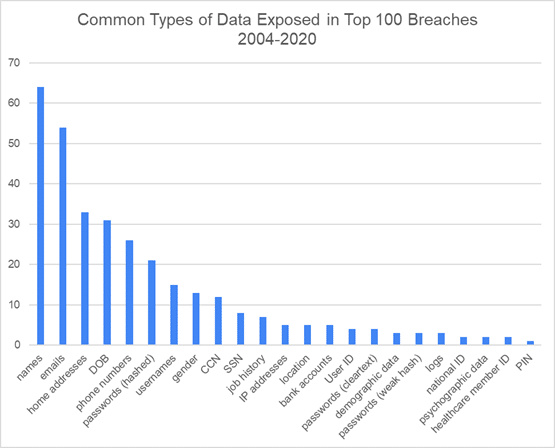
The vast majority of breaches expose names and email addresses. Overall the top five types of records that were exposed in the incidents we examined were:
- Names (appeared in 64% of breaches)
- Email addresses (appeared in 54% of breaches)
- Home addresses (appeared in 33% of breaches)
- Dates of births or DOB (appeared in 31% of breaches)
- Phone numbers (appeared in 26% of breaches)
If we combine password hashes with clear text passwords into one category, then passwords would make the fifth spot, above phone numbers (appeared in 30% of breaches). For the most part, passwords exposed in breaches were hashed with Bcrypt or another secure algorithm. However, in some cases, especially in older breaches, passwords were encrypted with weaker algorithms like SHA-1 or MD5. In other cases, passwords were simply exposed in cleartext.
Data Breach Breakdown by Industry
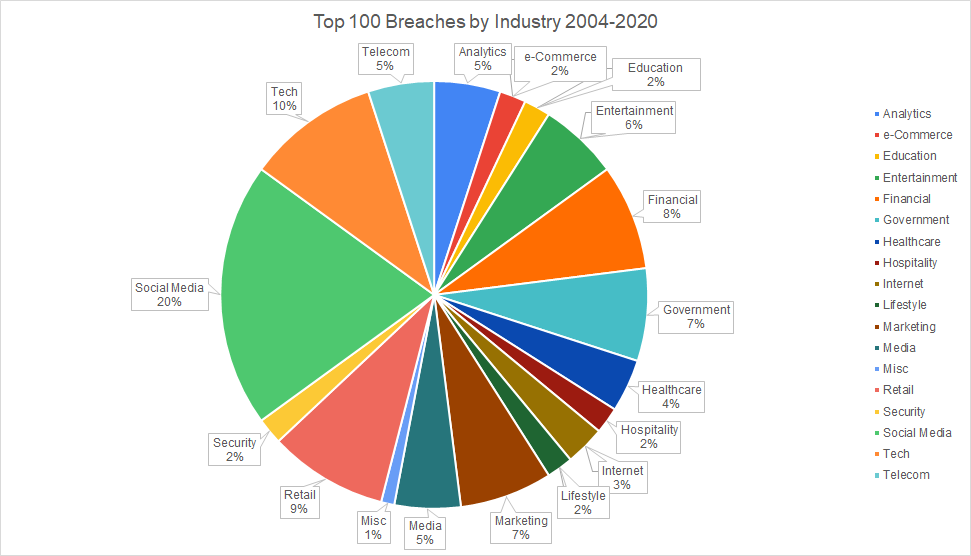
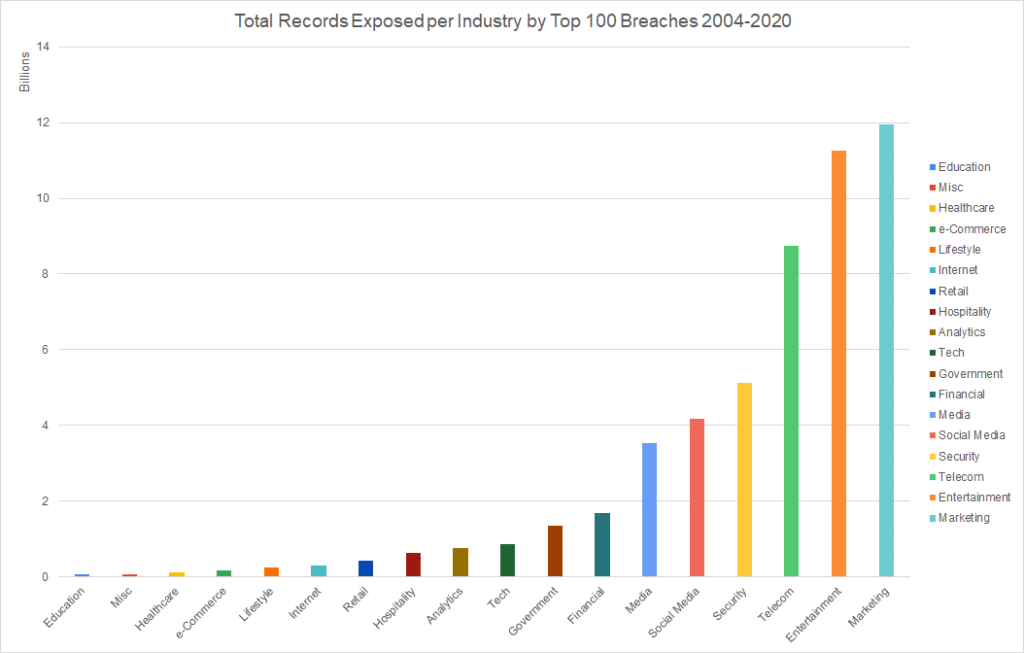
We looked at mega-breach impact across industries and found that social media data breaches, specifically those involving Facebook were the most common. Social media breaches were 20% of mega-breaches and Facebook or developers of Facebook applications made up 7% of mega-breaches. Other industries by size include:
- Technology service providers, which were the second most impacted industry. This included firms like Adobe, Uber, and Microsoft (10% of data breaches). While these breaches were large, proportionally this industry exposed just under 2% of the records in the dataset.
- Retail companies like TJX and Target. These were more prominent towards the earlier half of the past decade and a half (9% of data breaches).
- Financial entities like Capital One, Korea Credit Bureau, Experian, and Equifax made up 8% of data breaches.
- Marketing firms, which included sales companies like Apollo as well as email marketing firms like Verifications.io and big data platforms like Oracle’s BlueKai made up 7% of data breaches. This industry had the largest total number of records impacted.
- Breaches of governmental agencies in the US and abroad also made up 7% of data breaches. These include the Philippines’ Commission on Elections (COMELEC), the UK Revenue and Customs as well as the US Office of Personnel Management.
- Entertainment broadly refers to a variety of firms producing content or events. We included gaming companies like Sony PlayStation (PSN), Zynga, as well as adult entertainment companies like CAM4 in this category. These made up 6% of data breaches. However, this industry had the second-highest total number of records impacted.
The remaining industries have relatively less representation in the dataset, though it’s worth calling attention to Telecom, Security, and Media given that these industries have a higher proportion of records exposed.
- Telecom made up 5% of data breaches in the list and consisted of companies like Verizon and T-Mobile. One breach from this industry, Advanced Info Service, exposed over 8 billion records.
- Media also made up 5% of data breaches. Three of these breaches came from Yahoo! which we included in this industry because of the company’s pivot from traditional internet search to media. One of these breaches was the infamous hack that exposed that data of Yahoo’s entire 3 billion users.
- Security only included 2% of data breaches. The first was the 2011 RSA hack believed to have been carried out by state actors. The second is a multibillion record leak by Keepnet Labs which had compiled records from old data breaches to study them. While this data had already been exposed before, it had never been collected and aggregated in this way. We counted this as a net new data leak as such a massive dataset would allow possible threat actors to more thoroughly cross-reference information on potential victims.
Analyzing the Causes of the Top 100 largest Data Breaches
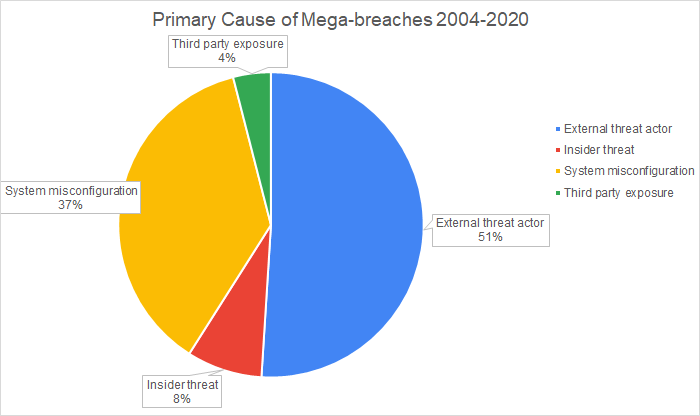
A little over half the top 100 data breaches were caused by an external threat actor. The second most common cause was a system misconfiguration. These are incidents that result in data becoming accessible over the internet without the involvement of a threat actor. Insider threat is the third most common cause. Finally, third party exposure is the fourth most common cause. Here, third party exposure means the data was exfiltrated directly from a business partner’s systems. Several of the mega-breaches that fall under the external threat actor category, such as the Target and Home Depot breaches, involve a threat actor staging an attack through vulnerabilities in a third-party system. These breaches utilized third party systems as a means to infiltrate the systems of the target organization as opposed to stealing data directly from a business partner.
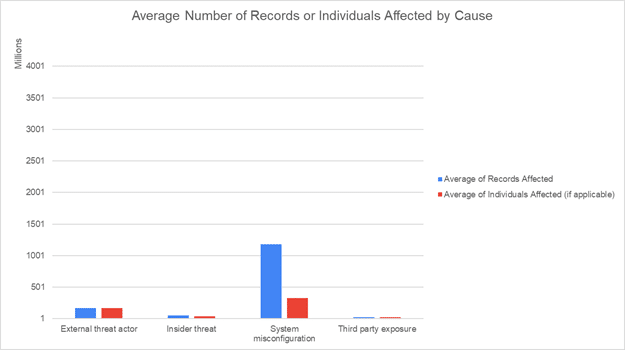
When looking at the size of mega-breaches, those that result from system misconfigurations tend to be much bigger. The average number of records exposed due to system misconfigurations is about seven times greater than exposures resulting from data breaches with external threat actors. This isn’t surprising as six of the seven billion-record mega-breaches are cloud misconfigurations.
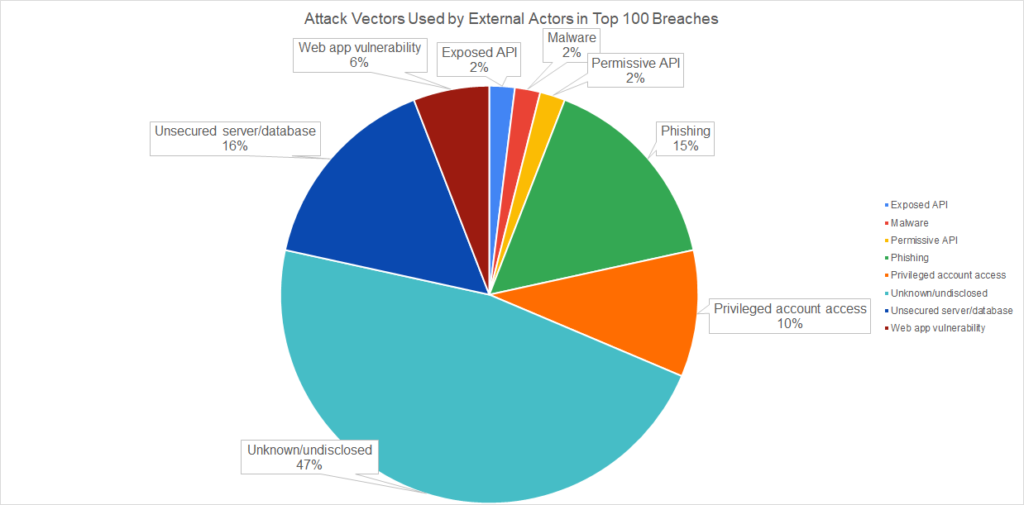
Since external threat actors represent the most common cause of mega-breaches involving a threat actor we looked at the most common ways they exfiltrated data. Unfortunately, for a lot of data breaches, forensic analysis was not provided making it hard to have a comprehensive picture of these incidents. For 48% of these data breaches, we don’t know how the threat actor got away with the data.
Among the data breaches where the attack vector is known, exploits of misconfigurations are among the most common attack vectors. These include:
- Unsecured servers or databases (15%)
- Web app vulnerabilities (6%)
- Exposed APIs (2%)
Permissive API is a special case in which an application is not technically insecure, but is designed in a way to allow for overly broad access to user data. The most high profile instance of a data breach involving this vector is Facebook’s Cambridge Analytica scandal.
Phishing is the second most common cause (15%) followed by privileged account access (10%). We used privileged account access to refer to incidents where an attacker is believed to have gained access to a privileged account by some means other than phishing. In many cases the exact cause wasn’t disclosed.
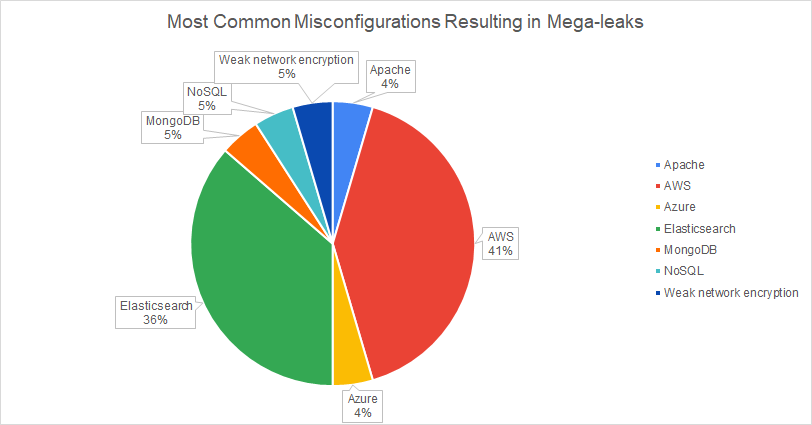
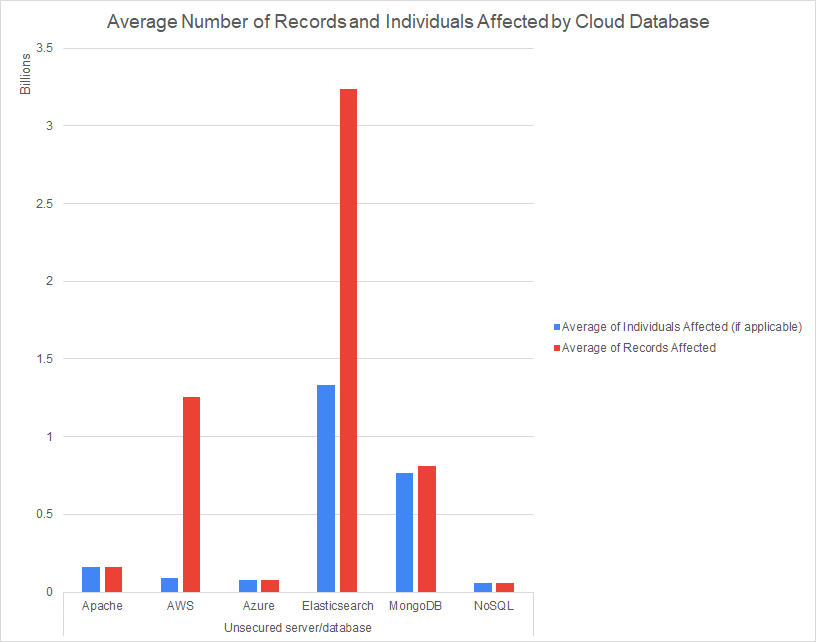
With unsecured databases resulting in a disproportionate amount of record exposures, we wanted to look into which systems were responsible for data leakage. Of the top 100 breaches we were able to tie 21% of them to a cloud database. AWS S3 (43%) and Elasticsearch (38%) respectively were the most common systems to result in mega-leaks. However, when it comes to the number of records exposed, Elasticsearch beats out AWS.
Costs and response time
Data on costs as well as time to discovery were fairly limited. We could only find costs for 26% of breaches in our dataset. We used news reports to determine costs, so the data we have tends to exclusively reflect expenses from settlements and lawsuits or legal fines as opposed to operational costs, losses, and security expenses. Thus, our cost estimates are likely on the conservative side.
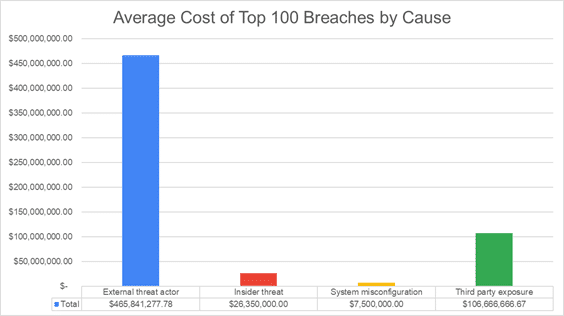
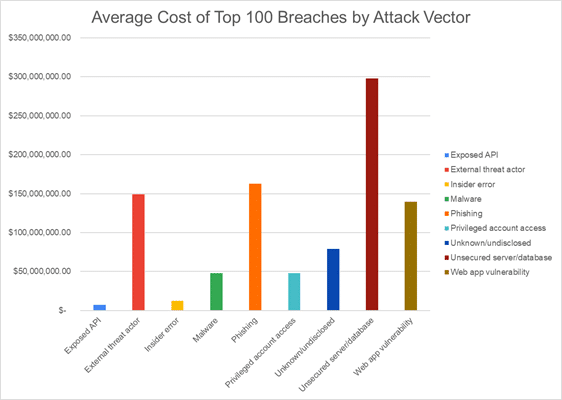
In the graphs above we assessed costs by cause, attack vector and by industry. In addition to being the most frequent breach cause, External threat actor is the most costly cause with an average cost of 465.8 million dollars. While system misconfigurations lead to the lowest cost on average, they’re the most likely to go unreported. Sometimes the news articles we reviewed for these incidents concluded with the company responsible for the misconfiguration failing to acknowledge they were aware of the problem. The story is somewhat different when we review the attack vectors used in breaches. Unsecured server/database and web app vulnerability are the first and third highest cost attack vectors in breaches and both result from misconfigurations. Together these make up 54% of the average total cost of breaches by attack vector.
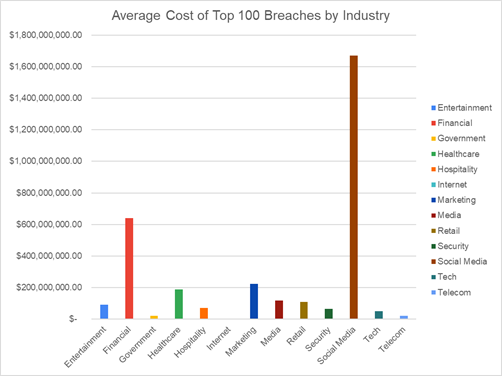
When we reviewed costs by industry, we unsurprisingly found social media companies had the highest costs on average. As we mentioned above, though, Facebook is overrepresented in the top 100 breaches. The 2018 Cambridge Analytica scandal specifically resulted in the company receiving a fine of 5 billion dollars. The Finance industry has the second highest costs, followed by the marketing industry.
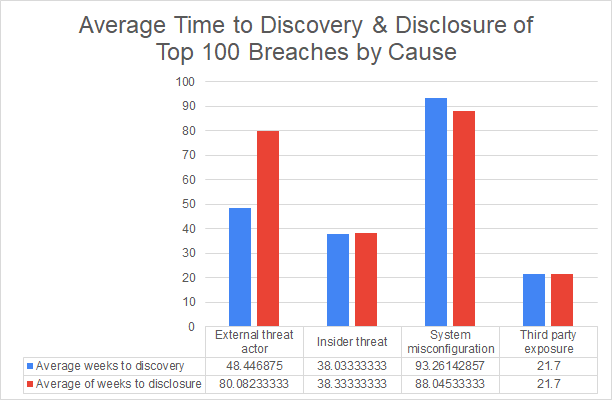
The last thing we looked at was time to discovery and time to disclosure for breaches. The latter refers to how long it takes for consumers to learn about the breach, usually from the affected organization, although sometimes news stories are the first to inform individuals. The former is a pretty common metric that reports like IBM’s Cost of a Data Breach Report provide (referred to there as "time to identify"). Of the breaches we looked at, we found details for time to discovery for 42% of them.
Time to discovery varied widely across the dataset, but we found on average leaks that resulted from a system misconfiguration, especially an unsecured database, log leak, or exposed API tended to take longer to discover, remediate and disclose. Breaches resulting from an external threat actor had the second highest time to discovery and time to disclosure on average. While breaches caused by external threat actors include misconfigurations like unsecured databases, discovery time was highest in breaches involving privileged account access (68 weeks and 4 days vs 22 weeks and 5 days for breaches where an external actor leveraged an unsecured database).
Conclusion
Unsurprisingly, many of these breaches drive home some of the most important lessons of the past decade, including:
- The risk of mega-leaks. We spoke before about the growth of mega-leaks. This is a relatively new trend, where a disproportionately small number of breaches are responsible for a majority of the records leaked in a year. Cloud data exposures aren’t new, but the high volumes of activity and data in the cloud today makes the cost of an error much greater.
- External threat actors seek out existing vulnerabilities. Based on our findings, about 24% of the attack vectors used by external threat actors could be considered vectors that leverage existing vulnerabilities. Defenders will need to continue developing ways to identify how vulnerabilities expand their attack surface.
- Mega-breach/leak events take a long time to find. The data we have on response time is limited, but nonetheless alarming. Almost 15% of data breaches took a year or more to discover, with 9% of data breaches taking anywhere between 2 to 8 years to discover. Even when excluding these extreme cases, the average time to discovery for breaches discovered in under a year was 13 weeks or about 3 months.
In order to address these issues, security teams will need to continue to invest in tools that will empower them. These include tools that provide better visibility into where in the cloud their data is stored as well as tools that can track and manage vulnerabilities in the systems and programs used by an organization. We go into more detail about this as well as detailed descriptions of the most common attack vectors in our Guide to Identifying and Securing PII Leakage in 2021.
We intend to revisit this data in future posts on data breaches, so subscribe to our newsletter below and be on the lookout for updates.