Learn how to implement data loss prevention (DLP) on Slack, and detect leakage of sensitive data across any Slack workspace with this free online guide. You can also download this guide for offline reading.
What is Data Loss Prevention (DLP)?
DLP ensures confidential or sensitive information (like credit card numbers, PII, intellectual property, trade secrets, API keys, and more) isn’t shared outside of your Slack environment by scanning for content within messages and files that break predefined policies.
DLP is important for both security and compliance reasons. With DLP in place, you’ll be able to:
- Protect users from accidentally or intentionally sharing sensitive information with a proactive approach to addressing data sprawl.
- Train and coach users on your data sharing policies, including specific guidelines for external communications when using Connect channels.
- Ensure compliance with regulatory requirements for HIPAA, PCI, GDPR, and more by protecting personal identifiers.
- Prevent toxic behavior such as profanity.
- Reduce manual time spent reviewing content shared in Slack while maintaining visibility into cross-organization communications.
What does DLP detect?
- DLP solutions should be equipped to scan a broad set of data types, including personally identifiable information (PII), protected health information (PHI), Finance and payment card information (PCI), Health, Networking, Credentials & Secrets (API keys, cryptographic keys), and more.
- Nightfall comes with pre-built detectors out of the box that cover a comprehensive set of data types, industries, and geographies.
- Nightfall provides the ability to add in custom detectors, rules, keywords, and regexes as well.
- Review our list of Detectors and learn more about them in our Help Center.
Does DLP scan files too?
- It shouldn't be assumed that all DLP can handle files. You’ll want a DLP solution that scans both files & messages.
- Nightfall supports a broad set of file types including but not limited to xls/xlsx, doc/docx, csv, plain text, ppt/pptx, PDF, HTML, and more.
What's the risk in Slack?
Most employees view private platforms and workplace tools like Slack as more secure, since they are invite-only, often causing them to drop their guard and share sensitive data in messages. In this way, productive employees become accidental insider threats, contributing to data exposure. Therefore, external SaaS apps with widespread adoption in the market have become a prime target for cyber threats and bad actors looking to find even one errant API key or password they can leverage to gain complete control of your most valuable digital assets. While access controls are also important to keep such channels private, it's best practice to also minimize sensitive data shared in SaaS and use a comprehensive approach to protect that data.
Does Slack have DLP functionality built-in?
Yes, after realizing how much market demand there was for DLP in Slack and the popularity of third-party apps like Nightfall to mitigate security risks in the app in recent years, Slack ultimately released their own native DLP tool to support users who want to "check the DLP box" for compliance purposes, but are not ready to commit to a purpose-built, third-party tool. How does Slack DLP stack up?
Like most native SaaS DLP solutions, Slack DLP relies primarily on regex patterns and simple matching rules that fall short in today's complex data security landscape. Crucially, Slack's regex-based approach lacks the sophistication to effectively detect and classify API keys, let alone distinguish between active and inactive ones–a critical security consideration.
Furthermore, its approach to Protected Health Information (PHI) detection relies on simplistic pattern matching, rather than employing sophisticated combination logic that considers both Personal Identifiable Information (PII) and PHI contexts together for more accurate detection. This creates significant security gaps for businesses dealing with varied national identifiers, or organizations handling unstructured sensitive data like API keys, passwords, protected health information, PII or other intellectual property. These types of sensitive data are common in modern businesses and will likely be missed by native DLP provided by Slack. The main reason? Slack is a communication platform designed to support collaboration, not security protocols.
Does DLP work on any Slack plan?
Many DLP solutions don’t support every Slack plan. Nightfall is the first DLP solution to support any Slack plan:
- Nightfall Pro is designed for Slack Free, Pro, and Business+ plans. You’ll be able to scan all public channels. Learn more.
- Nightfall Enterprise is designed for Slack Enterprise plans. You’ll be able to scan the entire Slack organization via Slack’s Discovery API. This includes all public & private channels, groups, and direct messages. Learn more.
Can DLP scan my entire Slack organization?
- Scanning your entire Slack organization means scanning all messages & files in all public, private, and shared channels, and all groups & DMs.
- If you wish to scan your entire Slack organization, the key things you’ll need are:
- A DLP solution that is a Slack Enterprise partner
- Access to the Slack Discovery API
- It’s important to note that only official Slack DLP partners like Nightfall are able to connect to Slack via their Discovery API.
- The Slack Discovery API is included in Slack Enterprise plans. Even if you have an Enterprise plan, the Discovery API may not be enabled by default. To enable the Discovery API or check if you have it enabled on your plan, contact Slack.
- See a full comparison of Nightfall Pro vs Enterprise.
How do I implement DLP on Slack?
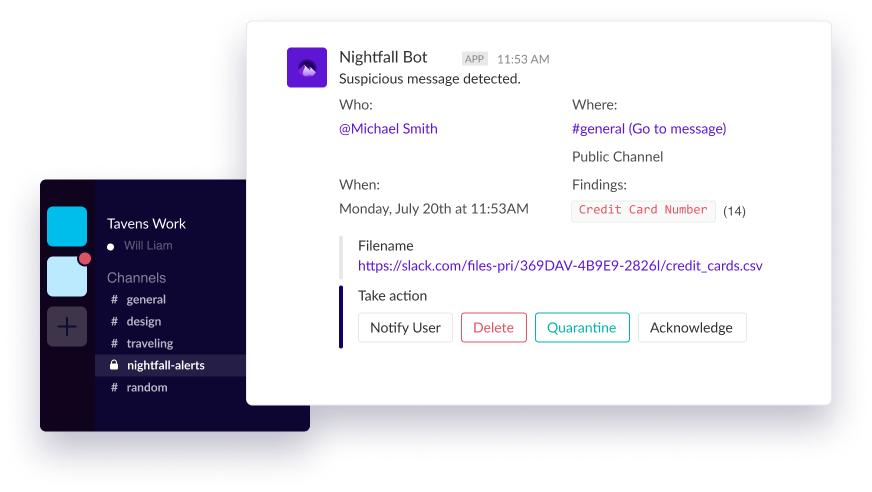
- You can implement DLP via third-party vendors that either (a) connect directly as a Slack bot (aka “cloud DLP” or “API-driven DLP”), (b) install as an endpoint agent on user devices (aka “endpoint DLP”), or (c) install as a network agent to scan for Slack-related traffic (aka “network DLP”).
- The Slack bot modality is recommended because it has no impact on end-users, will work regardless of the end-user’s device or network, has the full context provided by the Slack API, and provides the ability to remediate sensitive data directly in Slack.
- Nightfall installs as a Slack bot, which means it can be added in seconds to your Slack account. Nightfall connects to Slack directly over Slack’s APIs.
- No additional set up, tuning, or installed agents are required. Schedule a demo.
What is Slack Connect?
- Slack Connect takes the concept of shared channels – channels where companies that interact frequently can collaborate – to the next level, offering a way for up to 20 organizations to work together over chat, and more. If your organization needs to share data frequently with those outside your organization, Slack Connect might be a good option.
- DLP becomes even more important when Slack Connect is enabled because users can now easily share confidential data with external users directly within Slack. External channels can be easily confused with internal ones. Accidental data sharing in shared channels is common.
What Slack plan do I have?
- You can find out which Slack plan you are on by navigating to your Admin settings at {your-Slack-subdomain}.slack.com/home
- Under the Billing tab, you’ll see the name of your plan. For example, you’ll see a line like: “Your workspace is is on the Pro plan.”
What is Slack DLP good for?
If you're just getting started on your SaaS DLP journey, native SaaS DLP like native Slack Data Loss Prevention can be a decent place to start. It's certainly better than nothing, because at least you can catch and remediate instances of data sprawl with regards to structured sensitive data. If you're concerned about what an attacker could find if they gain unauthorized access, or if you're under strict compliance requirements, this won't be enough in the long run. You'll still have significant blind spots. Still, it's important to start somewhere in strengthening your security posture.
Core Slack DLP Features
Slack DLP scans Slack messages, text-based files, and canvases shared by your organization's Slack users to help identify structured content (bank routing numbers, CCNs, etc.) that violates company policy for data security. The tool also allows you to build custom rules against which it will provide real-time alerts, based on what it is able to find.
Slack DLP Detection Capabilities
Slack uses regular expressions and pattern matching to discover sensitive data, which is why it does a good job with structured, predictable formats. If you set the tool to alert on any other data sets, such as PII, API keys, PHI, etc., your security team is likely to be inundated with false positives, resulting in alert fatigue that can lead to overlooked potential threats later. Without custom detectors and the ability to handle unstructured data well, however, it's likely to miss a lot of what you need to secure.
Nightfall is a Slack DLP partner.
Here is a list of capabilities, features, and downsides to help you evaluate:
Is Slack Enterprise DLP good for comprehensive data loss prevention?
Slack Enterprise DLP Detection:
- Basic rule-based detection using regex patterns and preconfigured rules
- Limited to scanning messages, and text-based files within Slack
- Preconfigured rules may miss targeted data and generate false positives
- No special handling for spreadsheets or complex file formats
- Not good for PII, PHI, IP, secrets, and other unstructured data
(If HIPAA compliance applies to your organization, it is important to note that a DLP solution is required to use Slack in a HIPAA-compliant way.)
Slack Enterprise DLP Coverage and Scoping
- Coverage limited to Slack workspace only
- Available only on Enterprise Grid subscription
- Basic use cases focused on content scanning
- Cannot protect data across broader enterprise cloud environment on apps such as Google Workspace, Atlassian, GitHub, M365, Salesforce, Zendesk and more.
Slack Enterprise DLP Ease of Use
- Basic interface for rule creation and management
- Manual setup process through organization settings
- Limited to dashboard alerts and Slackbot notifications
- No automated annotation experience for administrators to reduce alert fatigue
Slack Enterprise DLP Remediation Capabilities:
- Limited remediation options: dashboard alert, user warning, or message hiding
- Basic automated actions when rules are violated
- Manual review required for hidden messages
- No automated remediation workflows
Slack Enterprise DLP User Training & Alert Interactions:
- Basic user notifications via Slackbot
- Limited user education or guidance
- No user feedback mechanism
Slack Enterprise DLP Architecture:
- No external API integration capabilities
- Operates within Slack's ecosystem only. No coverage on other SaaS apps, GenAI apps.
- No integration with identity provider platforms such as Okta, Google Directory, or Entra ID.
Slack Enterprise DLP Security Workflow Integration:
- No integration with external security, SIEM/SOAR tools
- Limited to Slack's internal security features
- Basic alerting through dashboard only
- No external reporting capabilities
What do mature security programs need to protect data in collaboration tools like Slack?
The average enterprise now has 75 or more security solutions to manage. When you're ready to protect against unauthorized file sharing, monitor export tools, meet regulatory compliance, implement on-the-job cybersecurity employee training, and do it all without interrupting employee productivity, it's time to look for a comprehensive cloud SaaS DLP tool like Nightfall AI.
This is what to look for in a SaaS DLP solution:
AI-Powered SaaS DLP Detection Capabilities
- State-of-the-art ML-trained detectors for discovering PII, PCI, PHI, passwords, API keys, and IP with high precision and recall
- Detects sensitive data in 100+ file types including docs, images, spreadsheets, HTML, PDFs, logs, and zip archives
- Leverages columnar information in spreadsheets and CSVs for improved accuracy
- Uses sophisticated PII, secrets and credentials and medical entity detection using LLM models
- Provides a wide range of pre-built detectors and policy templates to help you affect security on day one
AI-Powered SaaS DLP Scope of Coverage
- Comprehensive coverage across SaaS apps, GenAI, email, and endpoints
- Supports multiple use cases: secret sprawl prevention, PII/PHI protection, data exfiltration prevention, data lineage and automated encryption
- Broad DLP coverage of business-critical SaaS apps including Slack, Microsoft 365, Google Workspace, Atlassian, Salesforce, Zendesk, GitHub and more
- Unified workflow across all platforms and applications including support for Mac and Windows
AI-Powered SaaS DLP Ease of Use
- Intuitive interface with simple policy creation and management, custom reporting and end-user workflows
- Quick setup process integrating with apps in minutes
- Clear, actionable alerts via multiple channels such as Slack, Webhooks, Jira, Teams
- Flexible policy management by users, groups, teams
- Integration with Okta, Google Directory, Entra ID and Slack directory for user, user group filtering
AI-Powered SaaS DLP Data Protection & Remediation Capabilities
- Flexible remediation options including automated, manual, and employee remediation
- Real-time, immediate, or delayed remediation actions. Flexibility as per organization’s security workflows.
- End-user remediation and inline coaching
- Customizable by SaaS, GenAI app and on a per policy basis.
AI-Powered SaaS DLP User Training & Alert Interactions
- No impact on end-user productivity
- Educational approach and in-the-moment coaching to improve security hygiene
- User involvement in the remediation process
- False positive reporting capability
AI-Powered SaaS DLP Architecture
- API-first, enterprise-scale architecture. Several customers using Nightfall DLP for Slack in production to scan tens of thousands of channels on a daily basis
- Low-latency detection and remediation at petabyte scale
- No impact on source applications
AI-Powered SaaS DLP Security Workflow Integration
- Seamless integration with SIEM and SOAR tools
- Webhooks and REST API support
- Compatible with enterprise security ecosystems
How do I implement DLP on Slack?
As a Slack partner, Nightfall encourages Slack users to take steps to protect sensitive data within the app. We're in the Slack marketplace, meaning Nightfall is on the list of approved third-party apps you can quickly integrate to improve security. Some smaller organizations choose start with Slack DLP, grow their security program a little bit, and ultimately make the switch to Nightfall AI using the Slack Marketplace when they are ready to add a true layer of security that prevents SaaS data leaks.
How do I get started?
- To get started with Nightfall, schedule a demo with our sales team or contact us directly at sales@nightfall.aiwith any questions.